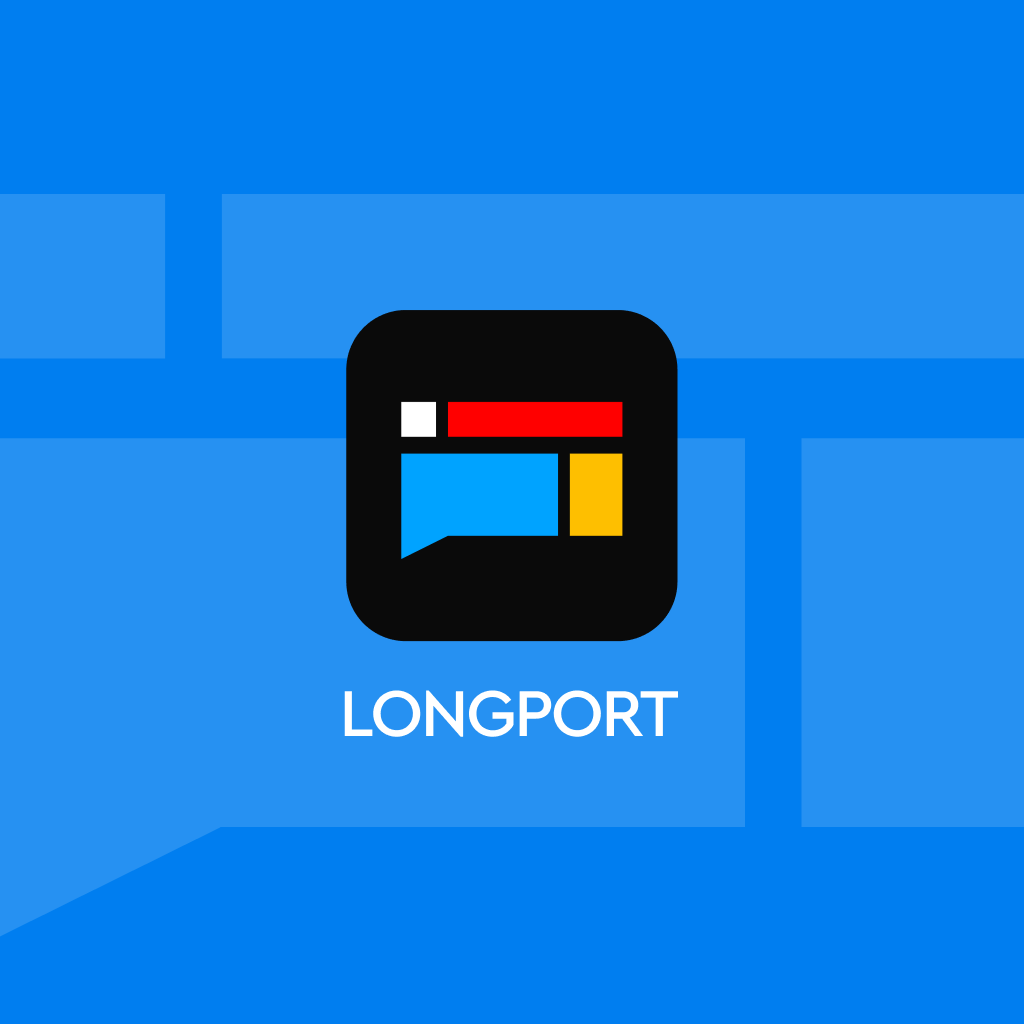
Jin Luyao: AI models have evolved from single-threaded to multi-threaded, which has changed the interaction process between humans and AI | Alpha Summit
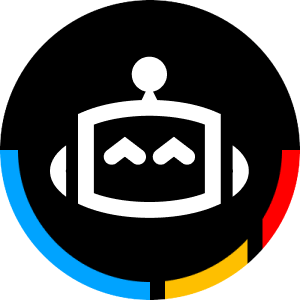
Jin Luyao stated that currently, everyone tends to regard large models as the capability itself, which allows for a variety of application forms packaged by AI models. Moreover, today's AI models have evolved into a multi-threaded process, beginning to draw inferences and answer questions that have never been encountered before, which changes the way humans interact with AI
On December 21, Jin Luyao, the product head of Alibaba Tongyi Lab, was a guest at the "Alpha Summit" co-hosted by Wall Street News and China Europe International Business School, where she analyzed and forecasted the evolution of AI applications and the driving forces behind them.
Here are some highlights from her speech:
- In the previous generation of AI models, large language models served as a foundation, while generating images and enhancing searches were plugins applied on top of the large models, which limited the forms of expression of AI models. Therefore, currently, people are more inclined to treat large models as the capability itself, allowing for a diverse range of application forms packaged by AI models.
- The earliest models were single-threaded, but now they have evolved into a multi-threaded process, enabling AI models to draw inferences and answer questions they have never encountered before. This has changed the interaction process between humans and AI. For example, in creating meeting minutes, it used to require many different modalities, but now there is an opportunity to integrate them, allowing AI to summarize, organize emails, and list schedules, becoming a true work-life assistant.
- The arrival of the large model era is beneficial for creative individuals, as AI models can assist humans in an efficient and novel way.
The following is a transcript of the discussion:
Hello everyone, my name is Jin Luyao, and I come from the Tongyi Product Department, where I am the product head. Today, I will share with you some successful experiences we have had since the establishment of Tongyi, of course, excluding some failures.
I just heard Teacher Chen in the previous session speak very well from an investor's perspective, which perfectly connects with my session. We can take a look at what is ready today and what lies behind it.
So, what lies behind it? I believe that all of you alumni should know better than I do that there is an invisible hand behind the economy, right? Today, the landing of AI applications or tools also exists with an invisible hand, which is our model capabilities. As Teacher Chen mentioned, what is ready in the market today? What is not ready? However, we often find that the process of searching for boundaries is very interesting.
For example, we find that the previous generation of models, whether in terms of text-based Q&A or the many people currently starting businesses with Xiaohongshu accounts and Douyin accounts to create a lot of content and original generation, often take the form of a chatbot, where you engage in dialogue with it. What does that process look like? Gradually, we call it a collaborative canvas, which may represent a newer form of creation, including methods. Let me break it down for you. The chatbot we see today is mainly just a chat box, including what has emerged later, including publicity Behind this framework is the process of each person accumulating their so-called knowledge during learning, which will help you every time you answer questions.
Today's model is based on the same concept. In August, we collaborated with the Olympic Committee to create an Olympic GPT, which often provided historical knowledge of the Olympics. What does this mean? For example, the original event commentators needed to search online for a lot of information to pick out the best result as a reference. Today, for large models, it means reading through all the results and determining which pieces of information complement each other to form a logical framework. Once organized, it resembles your secret technique, providing you with a specific result that you can use directly. So what is search? Essentially, when you use Baidu, you are also finding the closest answer to what you want. Today, it is a process of reading, learning, and summarizing knowledge, so it is more about the exchange of knowledge. We will see how far this interactive form can expand, similar to what we are doing with the digital persona of Li Bai.
I also noticed that Teacher Chen mentioned correct AI. Correct AI, including Mini Max, often operates in a way that uses a digital persona to express optimal answers or knowledge. In the first generation of models, which we refer to as the previous generation, this was basically the way to answer questions from the audience or explore answers you wanted.
Many related products will gradually emerge in the market. For example, why did correct AI emerge early on? Because it can generate a lot of dialogues during interactions with people, helping you with assistance. What does our Li Bai digital persona do? It engages with elementary school students in rural areas of Guizhou, allowing them to ask questions and recite Li Bai's Tang poems or answer questions about his life and contributions. Recently, we also collaborated with the Nanjing Museum on reviving cultural relics and answering historical stories, which has led to some innovations.
So when we talk about whether the model is ready today, we need to address a question: what is it that is definitely ready today? This is the first question we will consider during the entrepreneurial phase of our large model. We will refer to it as what kind of generation this is. What are the pain points in the market? Where are the boundaries of the model? We hope that by releasing such interactive products, everyone can use them to support their careers and industries.
As the model evolves, what I am presenting now is the architecture of the previous generation model when applied. You will see that today’s large language model serves as its foundation, with all the foundational elements hidden behind this framework Then some of the capabilities of the models we refer to, including image processing, as well as some image generation and enhancement searches, are actually implemented in the form of plugins and applied on top of large models.
This can lead to a negative consequence, which is that it limits the forms of expression today and also restricts the initial threshold for everyone using it. Therefore, when we are starting up in this generation, especially in application entrepreneurship, we tend to regard today's large models as what? As atomic capabilities themselves, for example, image generation is one capability. For instance, text question-answering is another capability, and when we open the camera for enhanced visual capabilities in a multimodal way, that is also a capability. Gradually, the forms it wraps will be diverse.
For example, today, there is Canvas, which perhaps everyone knows or doesn't know; it is a product I really love. What would it look like when it transforms from the limited dialogue with large models into something else? For example, I once heard about a student from China Europe International Business School who might have read 16 papers, and the teacher assigned him a paper saying that today is Sunday, and he needs to submit it by Wednesday. Such an assignment is relatively difficult to complete, but just as a joke, this student uploaded those dozen papers directly to ChatGPT. Originally, the format could only provide a question-and-answer interaction, asking what those 12 papers were about and summarizing them. Today, if we use this interactive format, you can say on the left, "Help me generate a new type of paper; I will roughly tell you my planning direction." Then? It will continue to answer you, indicating which relevant contents are from those 12 papers, and automatically respond on the right. You might say, "Oh, this paragraph I think is far from enough; I hope it can be polished again." You can select the content you want to refine, and during its reading of those 12 papers, it can perform more detailed processing, including summarizing paragraphs, helping you extract them, and then supplementing them into that paragraph of the paper. Isn't this a faster creative process?
I believe through this process, everyone should be able to think that today, the entrepreneurship of writers or media might undergo a transformation. For instance, we at Tongyi have been researching how we can help journalists from Zhejiang Daily write their editorials and news more efficiently, as well as assist every ordinary user in obtaining information in their areas of interest. Today, there might have been a total of 25 events; is it possible for me to spend 10 minutes reading them all when I wake up every morning? How are these processes creatively generated? It is more through such an application architecture that these model capabilities are relatively ready. The next step is that, as mentioned earlier by Professor Lang Chen, O3 was released this morning, and previously, the more ready O1 model was generated. What kind of changes will it bring to our lives? Then I will still use ChatGPT as an example. The two generations of models from OpenAI actually reflect the characteristics of the paths we choose when developing models in this industry.
For example, it focuses more on multimodal capabilities, but in its earlier generation, it did not possess many emergent reasoning capabilities. What does this mean in plain language? It was unable to generalize from one example to another. In the era of OE, it was able to achieve this, and at the same time, its logical reasoning abilities could gradually handle math problems, process Olympiad information, and perform a lot of coding. So where do we find its distinction? Initially, there were some models, meaning that models like this one were operating in a single-threaded manner. We find that when we do something, its modality and memory, including its reflection, are often bound to the same task flow. Humans, however, do not consider problems in such a way; we actually think about both logical aspects and emotional aspects simultaneously, and we may bring in fragments of past conversations between you and me.
This is actually a multi-threaded process, not a single-threaded one. Therefore, you will find that the O generation of models, whether O1, O2, or O3, represents a tendency in this part of logical reasoning towards different mediums or different modalities that provide feedback, allowing for a comprehensive processing that leads to a phenomenon of generalization. This is very similar to how we often evaluate an employee in a company: if I teach you something today, how many times do I need to teach it before you learn it? If you learn it after one teaching, I would say you are very smart. This is also why we find that at the multi-threaded stage, we become highly individualized, and people discover that models are becoming increasingly intelligent. Because they have the ability to solve more problems and tackle more complex tasks, even this morning with O3, we saw the most exciting point: it can even answer questions it has never encountered before. What does this resemble? It is akin to a scientist today trying to solve something that has not been solved by others; they have the means to do so, perhaps not perfectly, but they can attempt it. This indicates that today's models are approaching a level of human intelligence, although there is still a long way to go in terms of application.
However, we find that based on OE's slow thinking and reasoning processes, it has precisely changed many existing interactions. For example, let's say we used to need to create meeting minutes; today, you need to utilize many different modalities. Now we have the opportunity to integrate them together, cascading end-to-end within the same model to accomplish what? First, during the meeting, it listens and helps you summarize based on each person's different voices. Then, during the process, you might interrupt and say, "I remember that last time in this meeting there was an action; does it exist in this one?" Well, recap, maybe he doesn't have it, but he can remind you that after the meeting, many people need to organize emails, including sorting out some to-do items, turning them into agendas, and even needing to mail them out.
It can end this process in a systematic way. When the play button or the recording button is paused, it will naturally generate an outline, which is the mind map we see in the diagram. It will gather various knowledge points that may have been mentioned during the meeting into different tags or different content for you to display. If you feel that you need to send this outline out in the form of an email, you can ask it to expand this outline into a paragraph written in a leadership tone or in an agenda format. This is all part of the interaction between the model and you during the process, so it can effectively assist everyone in their work or in recording, and even at the end, it has a crucial capability, which is something we have been looking at recently as a very promising first-generation user product: it can help everyone take class notes. You can take photos while listening and insert them into the previous summary. When it helps you organize into an agenda, if you feel that there is a segment of knowledge points that you haven't understood clearly, you can ask it to repeat. You can select it, and it will take you to that chapter, help you expand on the knowledge points, and even help you search online to bring in knowledge that you didn't hear in class. This greatly lowers the barrier for us to learn knowledge.
Then we can see another diagram, where we have created a cascading model. This looks like a Douyin image, with a layer overlay, but it is actually a translation. In August of this year, we first showcased this product at Yunqi, which means that whether in multinational meetings or in many situations today, such as watching American dramas that may be raw and have no subtitles. Originally, if you wanted to do simultaneous translation or subtitle work, you might have to wait two days for simultaneous translation, as it might only translate the next sentence after hearing a complete sentence. Today, our translation model, through the connection of the multi-threaded model mentioned earlier, can achieve millisecond-level output, meaning that as soon as the first English word is spoken, the translation appears. When we presented this at Yunqi, the businesswomen from Yiwu found it incredibly exciting, and basically every businesswoman expressed that they definitely wanted to buy this model to facilitate their overseas business. So, as Teacher Chen mentioned, I think in terms of P and B, this year is very ready. There are many ToB tasks that can be done, and as long as everyone has imagination, this tool's innovation is relatively ready. This also tells us something: originally, in the early days of model entrepreneurship, we called it PMF, but perhaps today PMF is far from enough Then you will find that the technical aspect has something new every month. And as you use different models each month, you will find that you are changing part of your views and concepts. But I always say that many times today, models are here to assist humans in learning, working, and living. They cannot replace you because we are still at level two, right? Basically, it has a certain level of professionalism, perhaps equivalent to a master's degree. Its breadth of knowledge is quite substantial; it's just that today's models possess the expertise of many master's graduates. At this point, they can assist you well in your work, helping you with various tasks, serving as life assistants, work assistants, and learning assistants.
So in this analysis, we look at where today's models can go. More often, we hope to balance the technical market with the user pain points. Are they aligned? If they are aligned, then it is a very good product, and it will definitely stand firm. Another point I want to raise, which our team has been discussing, is that today's model entrepreneurship or the arrival of large models benefits a certain type of person. They must be very creative and eager to change the previously mundane aspects of life, hoping to have a more efficient or innovative way to assist them.
For example, we have recently seen many online tools where people come to Tongyi to create agendas, and there are some interesting capabilities related to interviewers. When the other party conducts an interview via video, the translator automatically provides an answer to a question that an operations expert should answer. Can we say this is a translation job? Although there are some tricky aspects here, it can be said that this is the beginning of an entrepreneurial journey and a landing of entrepreneurship. So it benefits all creative individuals, all liberal arts students, and anyone who has their own ideas in creative expression. For instance, our Wanxiang platform has recently been upgrading the X model, which is entirely a different technology stack from our current large text models. As you mentioned, Teacher Chen, it relates to an understanding of the objective physical world, and it is two separate systems: one represents your eyes, and the other perhaps represents your mouth or ears. The capabilities of these different models can actually help everyone in different ways. For example, many have seen the collaboration between Meta and ChatGPT on that pair of glasses, which often represents technological innovation. It doesn't only happen within the screen; it can also change every piece of hardware. So today’s glasses are like this; how can today’s necklaces not be like this? Therefore, there can be even more innovations to change things that you feel are impossible today Perhaps the model can be done today, so my sharing ends here. Thank you, everyone